This data was originally featured in the June 28, 2023 newsletter found here: https://www.trustinsights.ai/blog/2023/06/inbox-insights-june-28-2023-monthly-reporting-part-4-common-crawl-in-ai/.
In this week’s Data Diaries, let’s answer a very common question about large language models, one that folks ask nearly all the time:
What are these models trained on?
When we talk about training a large language model, everything from the open source projects like LLaMa to big services like ChatGPT’s GPT-4, we’re talking about the ingestion of trillions of words from content all over the place. One of the most commonly cited sources across models is something called Common Crawl. What is it?
Common Crawl is a non-profit organization that crawls and archives the web. They’ve got 7 years worth of the web indexed and make it available to the general public for free. What’s in this archive? Well… pretty much everything that’s open on the web and permitted to be crawled and indexed.
As of the most recent crawl, there are over 88 million unique domains in the index comprising over 50 billion pages of text. It’s 6.4 petabytes of data.
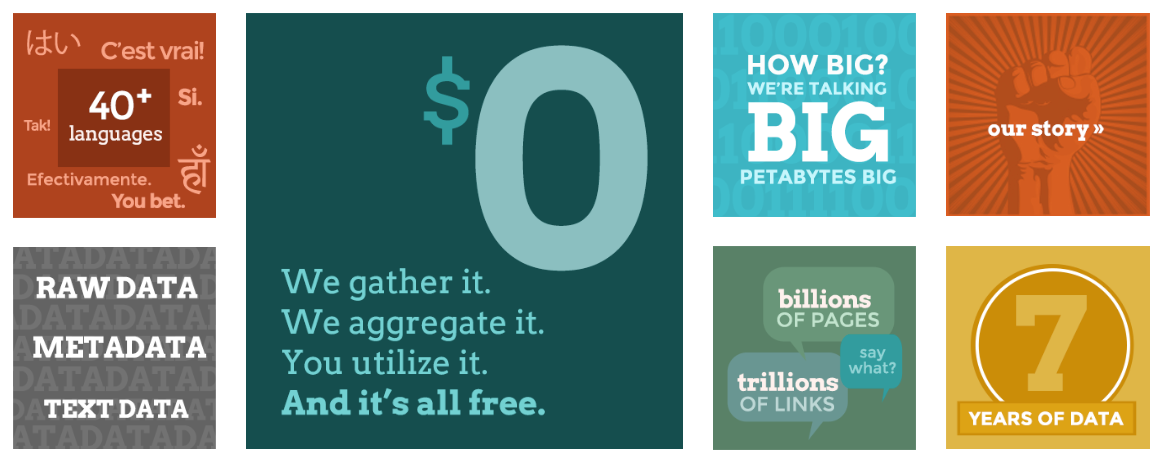
How large is a petabyte? If you take the average high-end laptop’s 1 TB hard drive, you’d need a thousand of them to equal 1 petabyte, so 6,400 laptops’ worth of storage. And bear in mind, this is just text. No images, no audio, no video, just cleaned text stored in machine-readable format.
Because this is a crawl of the open web, there’s a lot of stuff in the Common Crawl that you wouldn’t necessarily want to train a machine on. For example, there are prominent hate groups’ content in the Common Crawl, as well as known misinformation and disinformation sites.
Why are these sites used in machine learning model building, when they are known to be problematic? For one simple reason: cost. Companies building large models today are unwilling to invest in the cost of excluding content, even when that content is known to be problematic. Instead, everything gets tossed in the blender for the models to learn from.
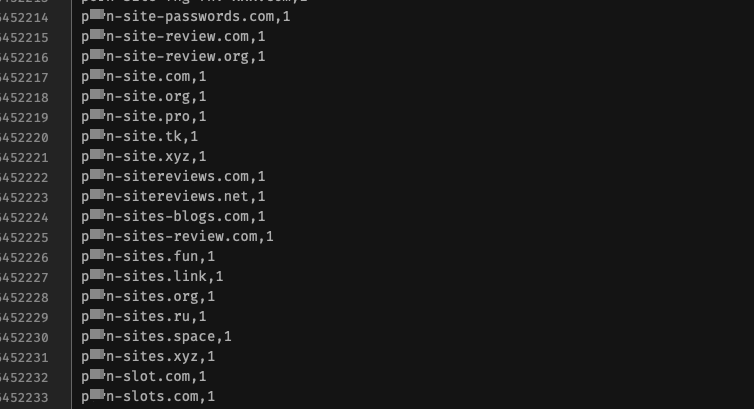
In some contexts, this is useful; a model cannot identify hate speech if it has no idea what hate speech is, so if you’re building an application to detect hate speech, you would need that in there. However, in the big generic models like GPT-4, this can also cause them to generate hate speech. For marketers and businesses, this certainly would be a problem.
What’s the solution? We are seeing companies and organizations start to build far more curated datasets, in part by taking Common Crawl and excluding obviously problematic content as well as low-rank content. For example, not every blog post on blogspot.com needs to be part of the training library, and certainly known problematic content can be excluded. As time goes by, expect to see more and more refined models that have no knowledge of problematic concepts to begin with, and those models will be best suited for commercial and business applications where mistakes would be completely unacceptable.
So what? As you embark on deploying generative AI solutions, particularly those based on large language models, realize that there’s more out there than just ChatGPT – vastly more. Be on the lookout for models that not only suit your specific use cases, but are free of the problems that earlier and larger models may have.
Need help with your marketing AI and analytics? |
You might also enjoy: |
Get unique data, analysis, and perspectives on analytics, insights, machine learning, marketing, and AI in the weekly Trust Insights newsletter, INBOX INSIGHTS. Subscribe now for free; new issues every Wednesday! |
Want to learn more about data, analytics, and insights? Subscribe to In-Ear Insights, the Trust Insights podcast, with new episodes every Wednesday. |