This data was originally featured in the June 5th, 2024 newsletter found here: INBOX INSIGHTS, JUNE 5, 2024: ROOT OF THE PROBLEM, GENERATIVE AI DATA
In this week’s Data Diaries, a bit of a reminder when it comes to generative AI. Right now, as business folks work on becoming proficient at generative AI, the quality and quantity of prompts you write confers advantage. Those people and companies who are great at this practice will generate better results faster than competitors. It’s one of the reasons we recommend our prompt frameworks like RACE and PARE to get you up to speed quickly.
That advantage is temporary as people skill up. So what’s an enduring advantage?
Unsurprisingly (especially given the name of this column), it’s the quality and quantity of your data.
Let’s walk through an example. Suppose you wanted to improve your social media marketing on a specific channel like LinkedIn. How would the average marketer go about doing this? You might fire up the large language model of your choice and tell it what you’re currently doing in broad strokes on LinkedIn, and ask for recommendations and best practices. This would generate fairly generic but likely helpful practices for LinkedIn marketing:
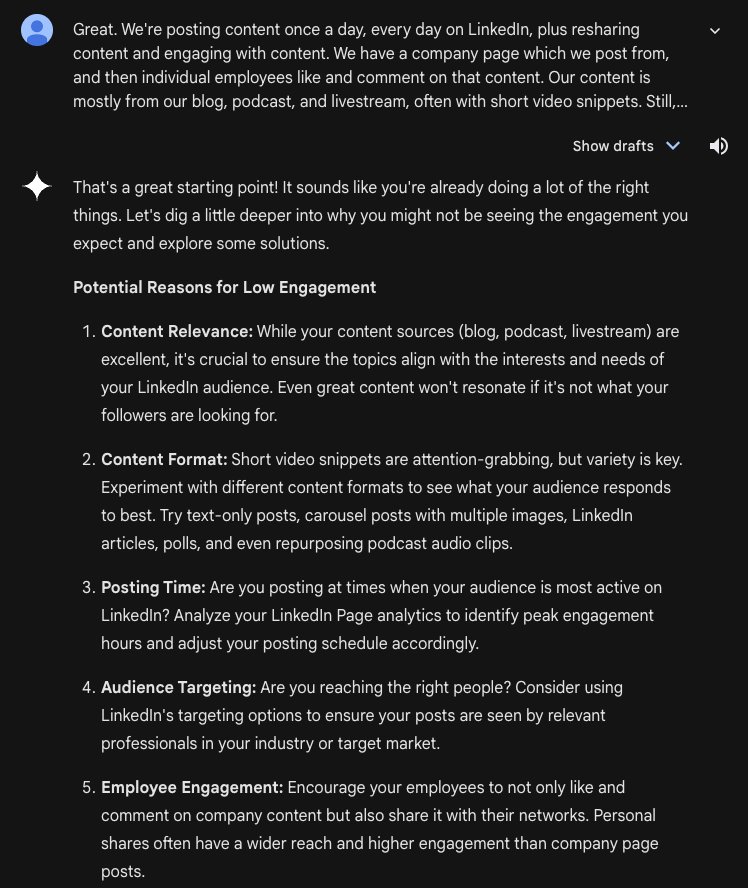
This is okay, and certainly if you’re not doing the basics, this is a solid standard operating procedure. However, this is also relatively plain; none of this information will confer any particular advantage to your company unless your competitors are in even worse shape.
What if you were to insert your data into the language model? How would that change things? I did exactly that, extracting data from our Agorapulse account for LinkedIn, and then asking the language model to come up with more specific recommendations:
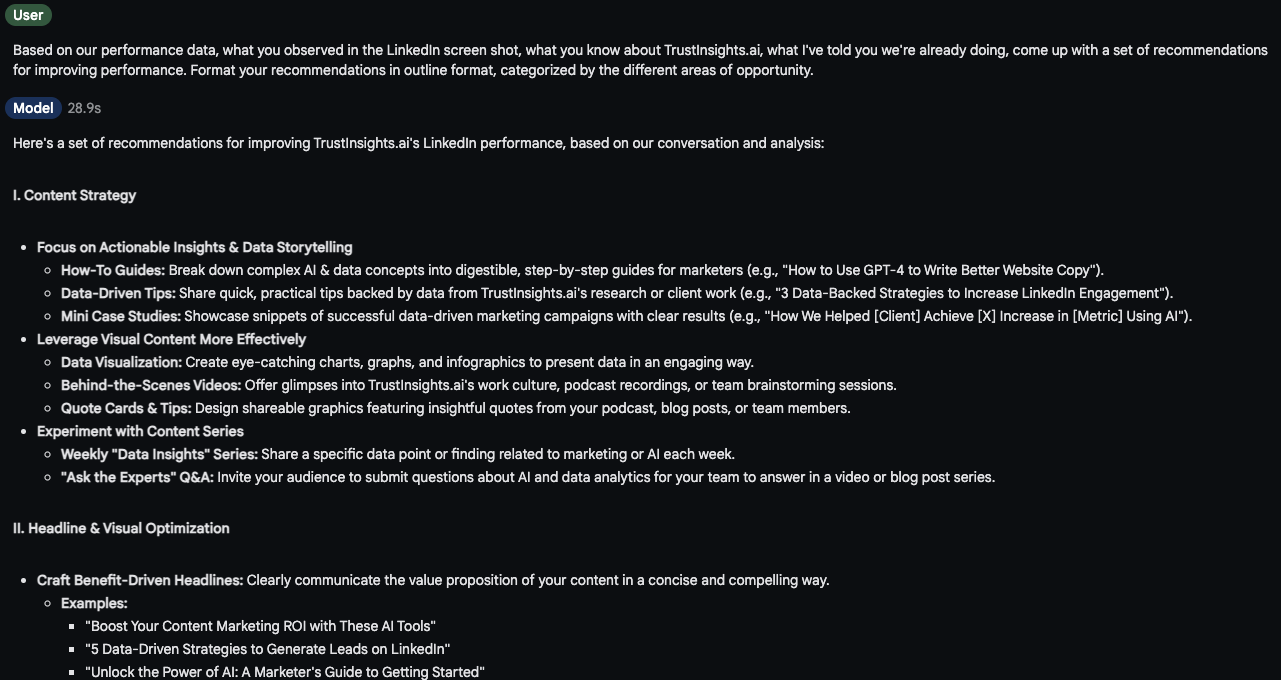
You can see we’ve gone from generic advice to advice specific to Trust Insights, which is a major improvement.
Now, what if you were to add some bespoke data, say… a comprehensive, data-driven guide to the LinkedIn algorithm? Over the weekend, I created an enhanced version of this guide. The guide itself is a useful read, but that’s not what I built it for. I didn’t build it for humans. I built it as data fodder for AI to use, a condensed summary of more than 70 blog posts, more than a dozen academic papers, etc. into a compressed format that AI can use as a reference. Suppose I add that in? How does that change our recommendations?
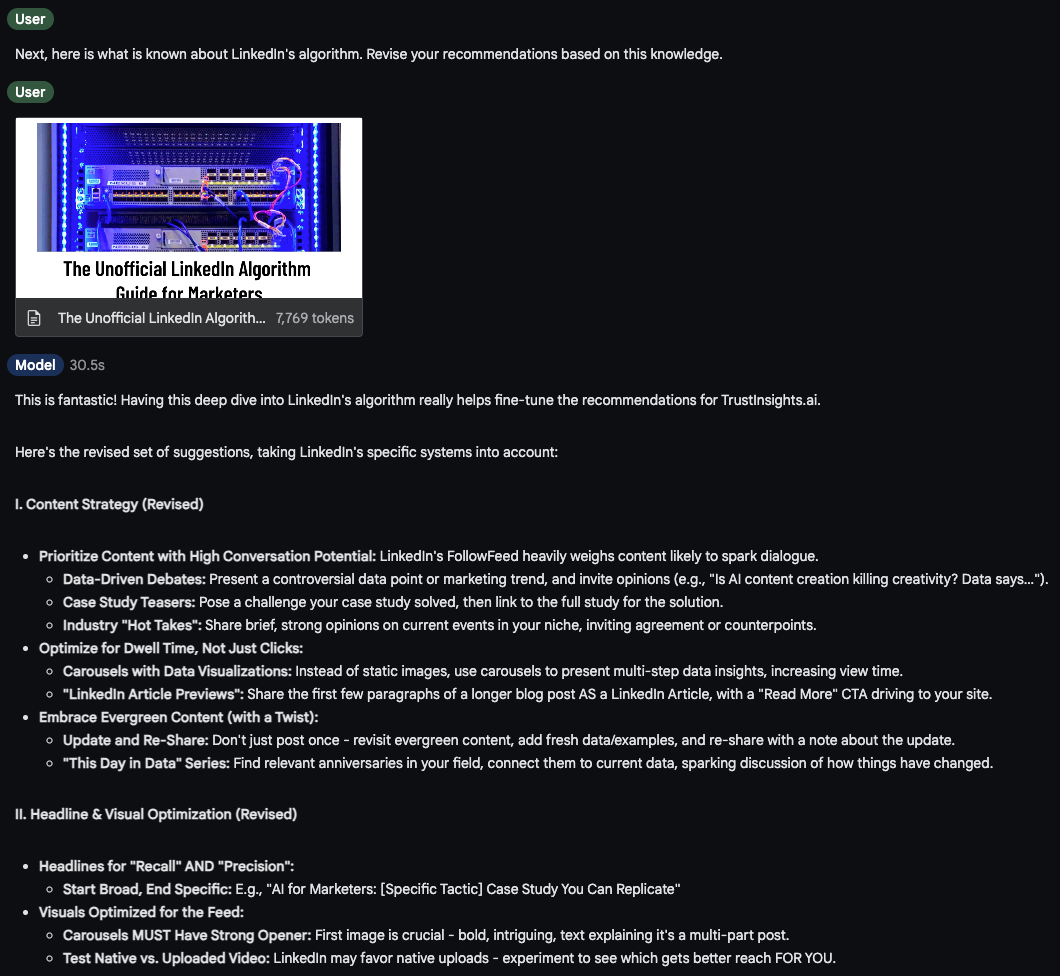
Now we’re cooking. We’ve got specific recommendations based on our data, plus recommendations tuned based on unique data that we built. It would be a strategic advantage if we weren’t giving it away, but you get the idea – if you had this data of your own, you could create recommendations and strategies that other companies couldn’t match because they wouldn’t have the data you have.
Even better, because we’re supplying so much data, the risk of hallucination (AI just making things up) goes down dramatically. One of the key rules of thumb with generative AI is that the more data you bring, the less it’s likely to make things up.
Your strategic advantage going forward in generative AI is based on the quality and quantity of data you have that you can provide to generative AI systems. Whoever has the most, best data will win. It’s critical that you not only get your own house in order in terms of data governance, but that you collect as much relevant, specific, bespoke data as you can.